Profile
I am a Ph.D Candidate at National University of Singapore (NUS), working with Prof. Feng Mengling. Before Ph.D, I graduated from NUS with a masterβs degree in electrical & computer engineering and did my research intern at IBM Research.
Research Interests: My research focuses on designing and applying AI models to address real-world healthcare challenges, with a long-term goal of building expert-level multimodal generalists to enhance clinical decision-making and patient care. My recent work concentrates on advancing multimodal large language models, leveraging their remarkable capacity for synergizing diverse modalities for reasoning and planning. Previously, I worked a lot on self-supervised learning models to improve label efficiency in the medical domain. I am also interested in time series modeling, and much of my work has involved time series data, as it is one of the most prevalent modalities in healthcare. I have published 10+ papers at the top international AI conferences and journals such as ICLR, ECCV, AAAI, Information Fusion, and TIST.
π₯ News
- [03. 2025] Our paper on empowering MLLM for grounded ECG understanding now available at arXiv.
- [06. 2024] Our paper on multi-stage contrastive learning is accepted in ECCV 2024.
- [06. 2024] I was awarded for the Graduate Student Research Award AY2023/2024!
- [01. 2024] Our paper on contrastive learning for time series applications is accepted in ICLR 2024.
- [10. 2023] Our survey on LLM for healthcare applications is accepted in Information Fusion.
- [05. 2022] Our paper about self-supervised learning for ECG is accepted in AAAI 2022.
π Publications
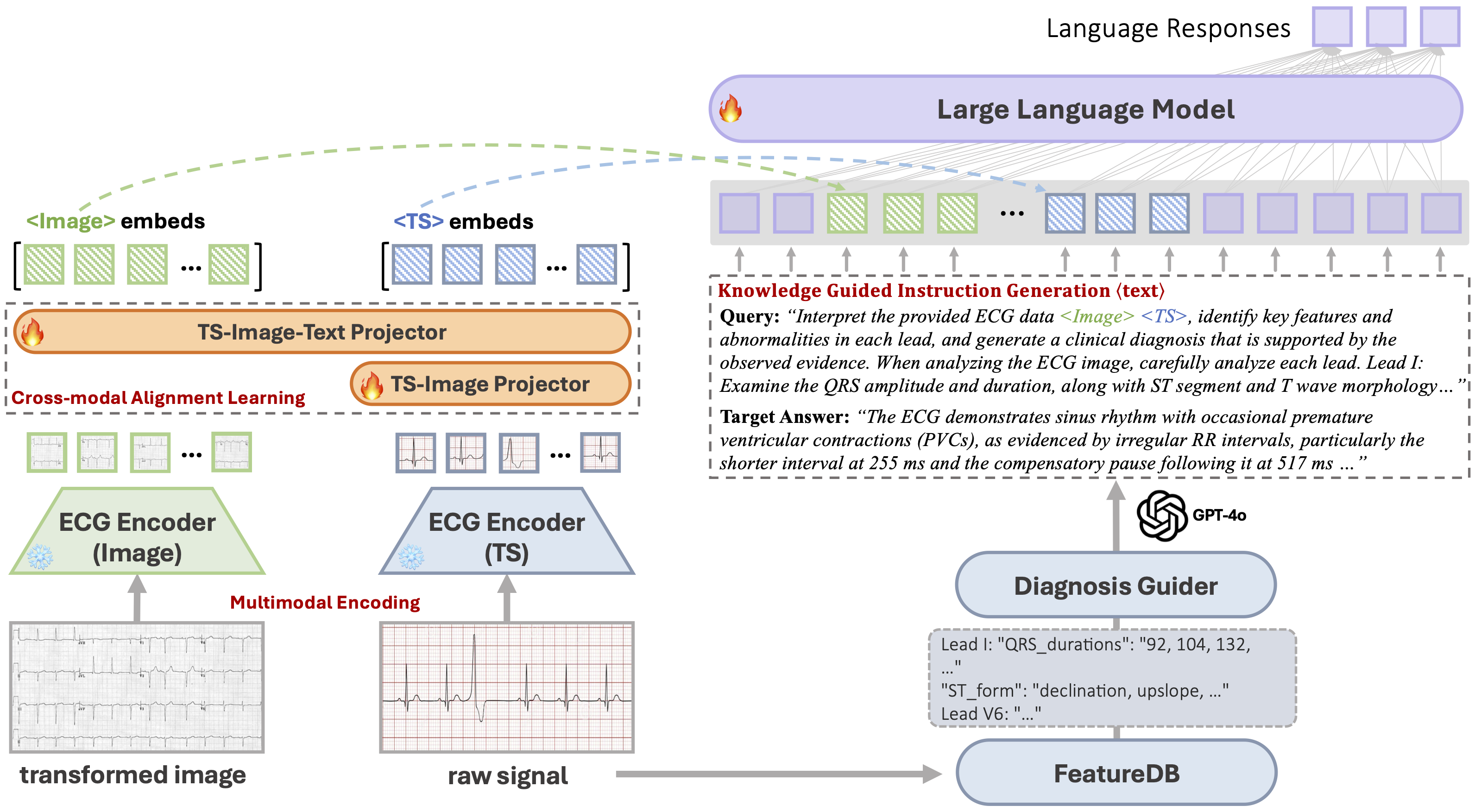
GEM: Empowering MLLM for Grounded ECG Understanding with Time Series and Images
Xiang Lan, Feng Wu, Kai He, Qinghao Zhao, Shenda Hong, Mengling Feng
- First Unified Multimodal ECG Model.
- First High-granularity ECG Grounding Dataset.
- Clinically Oriented Diagnostic System.
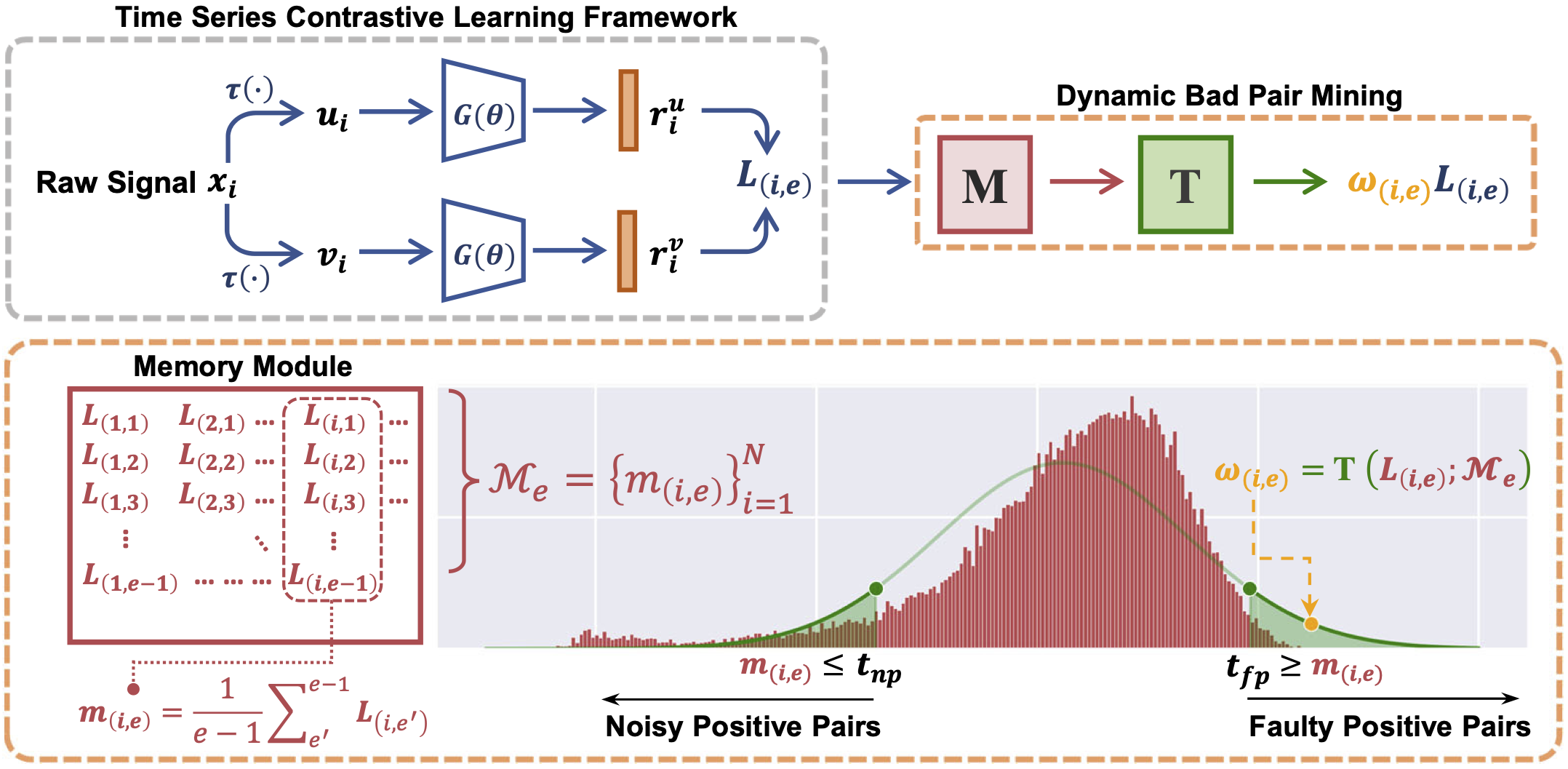
Towards Enhancing Time Series Contrastive Learning: A Dynamic Bad Pair Mining Approach
Xiang Lan, Hanshu Yan, Shenda Hong, Mengling Feng
- First study to investigate the bad positive pair problem exists in time series contrastive learning.
- A simple yet effective algorithm designed as a lightweight plug-in.
- Enhancing the performance of existing state-of-the-art methods.
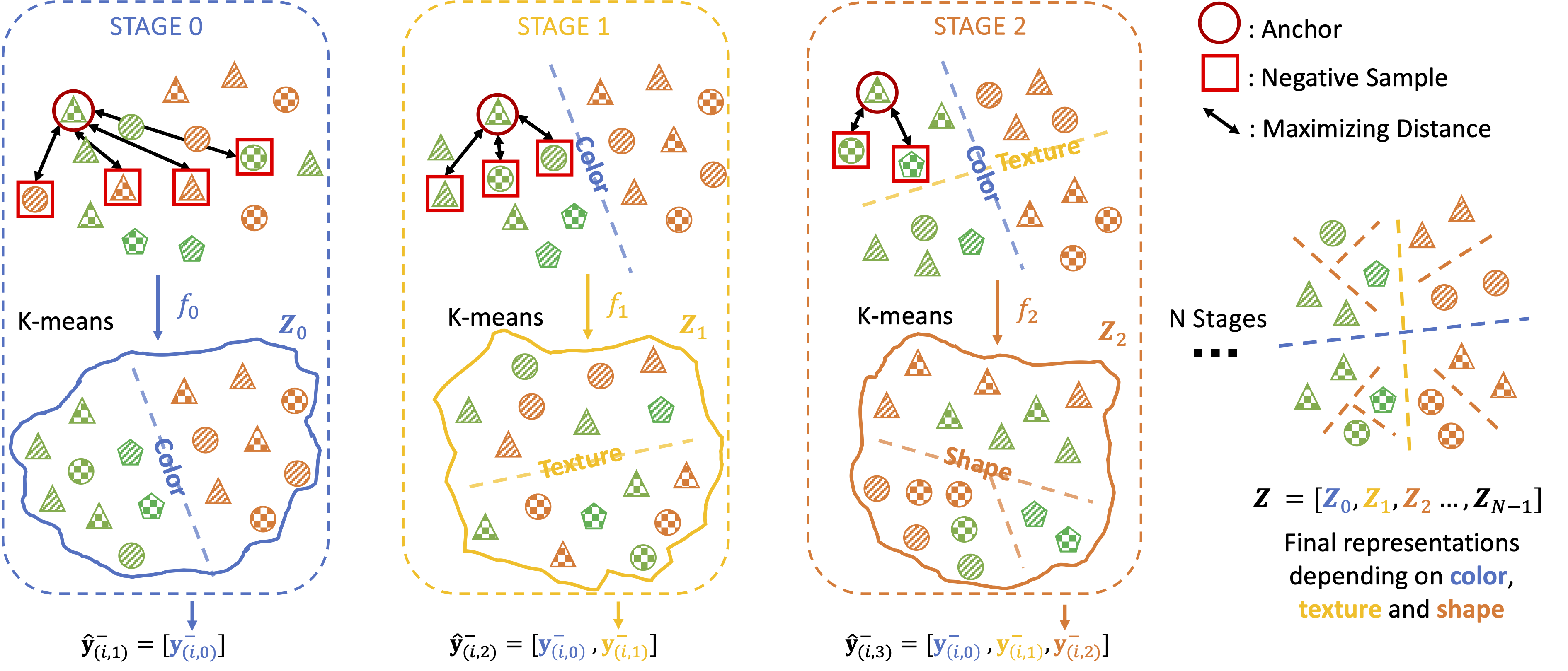
Learning the Unlearned: Mitigating Feature Suppression in Contrastive Learning
Jihai Zhang*, Xiang Lan*, Xiaoye Qu, Yu Cheng, Mengling Feng, Bryan Hooi
(*co-first author)
- First multistage contrastive learning framework.
- First work to discuss and address feature suppression in both unimodal and multimodal contrastive learning.
- Adaptable to various contrastive learning settings.
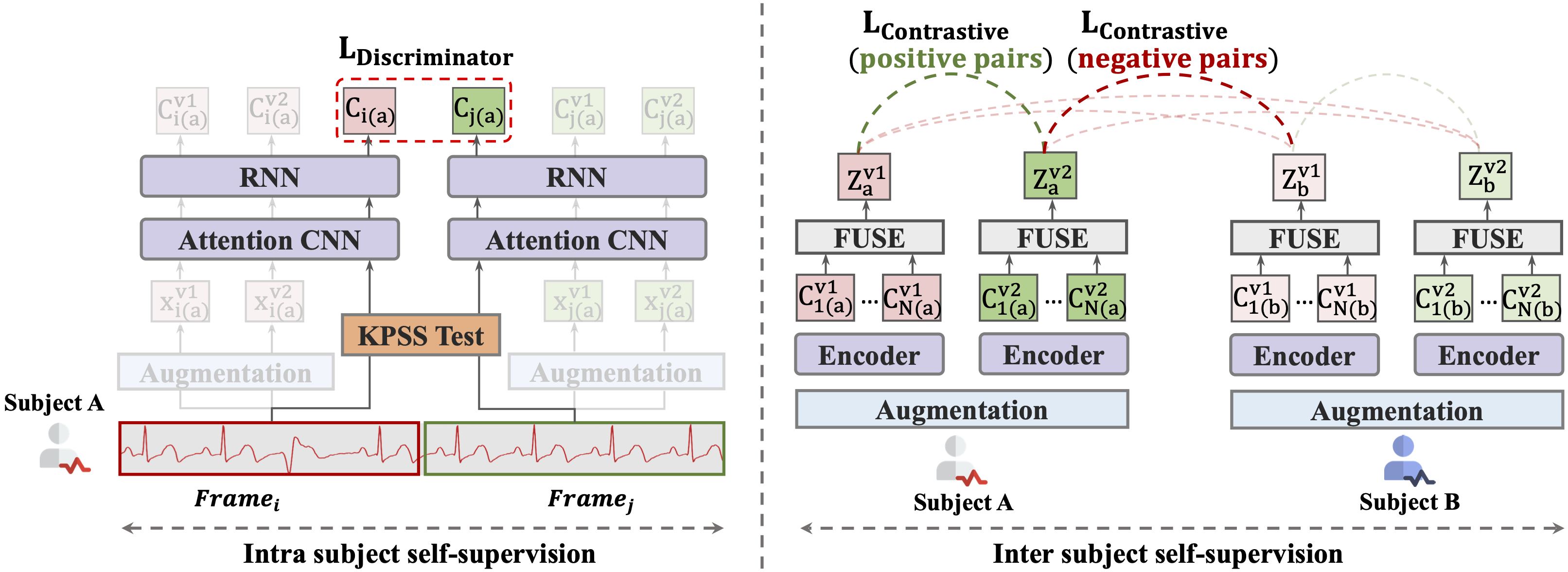
Intra-Inter Subject Self-Supervised Learning for Multivariate Cardiac Signals
Xiang Lan, Dianwen Ng, Shenda Hong, Mengling Feng
- First work that integrates medical knowledge into self-supervision to boost the performance of cardiac arrhythmias diagnosis.
- Novel intra and inter subject self-supervision mechanism.
- State-of-the-art performance.
-
Kai He, Rui Mao, Qika Lin, Yucheng Ruan, Xiang Lan, Mengling Feng, Erik Cambria
Information Fusion
-
Language Modeling on Tabular Data: A Survey of Foundations, Techniques and Evolution
Yucheng Ruan*, Xiang Lan*, Jingying Ma, Yizhi Dong, Kai He, Mengling Feng
preprint
(*co-first author) -
Hairil Rizal Abdullah, Daniel Yan Zheng Lim, Yuhe Ke, Nur Nasyitah Mohamed Salim, Xiang Lan, Yizhi Dong, Mengling Feng
Korean Journal of Anesthesiology
-
Yucheng Ruan*, Xiang Lan*, Daniel J Tan, Hairil Rizal Abdullah, Mengling Feng
preprint
(*co-first author) -
Federated Learning for Electronic Health Records
Trung Kien Dang*, Xiang Lan*, Jianshu Weng, Mengling Feng
ACM Transactions on Intelligent Systems and Technology
(*co-first author) -
Xiang Lan*, Dianwen Ng*, Yi Liu, Jiongzhou Liu, Fan Xu, Cheng He and Mengling Feng
IJCNN 2021
-
Zhaowei Zhu*, Xiang Lan*, Tingting Zhao, Yangming Guo, Pipin Kojodjojo, Zhuoyang Xu, Zhuo Liu, Siqi Liu, Han Wang, Xingzhi Sun, Mengling Feng
Physiological Measurement
(*co-first author) -
Dianwen Ng, Xiang Lan, Melissa Min-Szu Yao, Wing P. Chan and Mengling Feng
Quantitative Imaging in Medicine and Surgery
π Honors and Awards
- 2024 Graduate Student Research Award, NUS
- 2020 Championship, SG Healthcare AI Datathon 2020
- 2020 2nd Runner-up, PhysioNet/Computing in Cardiology Challenge 2020
π Educations
- 2021.07 - now, Doctor of Philosophy, National University of Singapore.
- 2018.07 - 2019.06, Master of Science, National University of Singapore.
- 2014.07 - 2018.06, Bachelor of Science, University of Electronic Science and Technology of China.
π Academic Services
I serve as a reviewer for ICLR, WWW, AAAI, KDD, TNNLS, TIST, Health Data Science.